Our approach at a glance
AI coaching leverages deep learning models to deliver data-driven, personalized coaching experiences that are scalable, unbiased, and psychologically safe.
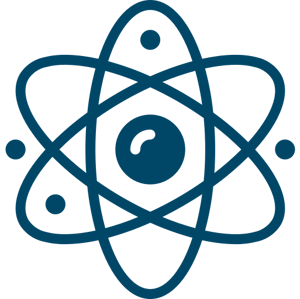
Retorio, founded at the Technical University of Munich and advanced at MIT and the University of Tokyo, leverages behavioral science and deep learning for personalized coaching.
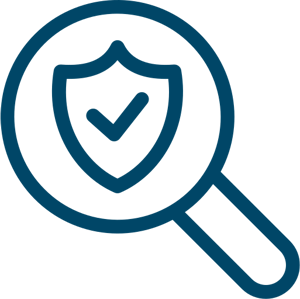
Retorio analyzes verbal, vocal, and visual cues using multimodal models and offers data-driven recommendations. Retorio emphasizes explainability and transparency in its AI models to avoid biases.
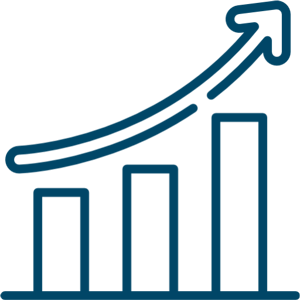
Retorio boosts learner improvement by 2-3% per session, with measurable business outcomes: a 5-15% increase in annual revenue, and cost reductions of up to 98% compared to conventional coaching.
AI coaching offers empirically validated business impact
Highly accepted by learners: AI coaching enjoys high voluntary participation rates (83% of learners) and high acceptance rates (84% of learners), significantly above conventional coaching formats.
Effectively closing skill gaps: High efficacy in reducing both implicit and explicit knowledge gaps. The average initial rate of gap reduction is observed at 3% over the first three coaching sessions and 2% across all sessions.
Offering a substantial business impact: Enterprises that improve the performance of their sales reps through AI coaching, see a 7% increase in revenue within four months.
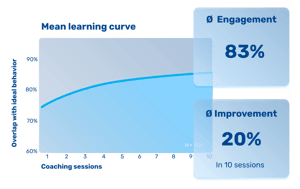
Combining cutting-edge AI technologies
Retorio's AI coaching platform integrates different sets of deep learning models. 93% of communication is non-verbal, only 7% is verbal. Retorio is multimodal and captures both verbal and non-verbal communication.
- 1. AI coaching generator
- 2. AI coach
- 3. Impact reporting
Retorio offers generative AI models that create coaching content based on your input and bring your content to life.
Purpose:
- Generate meaningful coaching content based on your input
- Translate goals into concrete winning behaviors
- Power dynamic simulations with virtual agents (avatars)
AI technologies:
- AI coaching generators (GANs for content creation)
- AI coaching simulators (LLMs for conversational AI)
- AI-enabled coaching libraries (expert-generated content)
Retorio's "brain" is its unique Behavioral Intelligence – a meta-model that analyzes learner videos and quantifies, predicts, and prescribes behavior.
Purpose:
- Quantify & interpret behavior from video data
- Identify implicit winning behaviors
- Generate feedback & recommendations
AI technologies:
- Recognition models (CNNs)
- Prediction models (CNNs, transformers)
- Benchmark models (LLMs, CNNs, or RNNs)
AI coaching impact models deliver insights into engagement, training, and business impact.
Purpose:
- Measure and visualize learning progress for learner cohorts
- Identify team, company, & industry-wide best practices
- Compare different coaching programs and predict coaching impact
AI technologies:
- Engagement analysis (descriptive models)
- Learning progress analysis (regression models)
- Business impact prediction (regression models)
Explainability and transparency
AI coaching must provide transparent and comprehensive model outputs. Retorio ensures this by presenting behavioral analysis results in an intuitive and easily understandable manner, fostering trust and acceptance among learners.
Transparency: Retorio offers transparency through visual elements like graphs and animations. Users can comprehend the AI coach’s analysis by connecting it with specific timestamps in the original video data. Additionally, Retorio employs text highlighting to further enhance transparency. This feature draws attention to keywords and phrases that have a substantial impact on the evaluation of communication.
Explainability: Retorio’s AI coach uses reasoning models (XAI), so that the AI provides textual explanations for its conclusions. This feature enhances the learners understanding of the recommendations and facilitates informed decision-making. Consequently, trust and confidence in the AI's capabilities are strengthened.
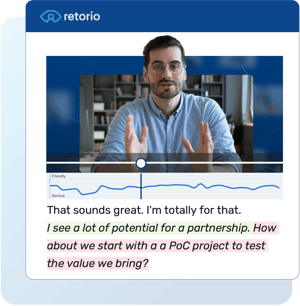
Powered by
Behavioral Intelligence
Retorio uses AI-powered Behavioral Intelligence to identify, predict and train winning behaviors in the context of job requirements, culture and industry.
While most coaching relies on self-reporting, our Behavioral Intelligence model focuses on actual observations by understanding behavioral cues such as facial expressions, gestures, tone of voice, and even language. It runs on scientifically validated models trained on millions of data points and is blind to factors like gender, age, and ethnicity.
Datasets and debiasing
Any AI is just as good as the data from which it learns. We have trained our AI on a broad data set ― millions of data points ― spanning a wide range of ages, sexes, and ethnicities. Retorio learns under human supervision and from scientifically audited datasets. While clients can configure Retorio’s models for their use cases with their data, Retorio’s base models do not learn from client data.
In order to avoid bias and generate representative results, our datasets represent behaviors and perceptions of people from diverse populations. We ensure that only factors that are within the control of the applicant are included in the results. We regularly test our models against large, scientifically sound datasets, such as UCLA's Fairface dataset, which contains approximately 100,000 individuals from different cultures, age groups, etc.
.png?width=300&name=Retorio-%20Recognition%20models%20%20(CNNs).png)
Quality control
A necessary condition for effective AI coaching is that the AI-supported algorithms can quantitatively determine behavioral characteristics with sufficient accuracy. Retorio places highest demands on the quality of AI models and algorithms. To ensure the highest quality standards for our AI models, we employ a combination of rigorous methodologies and best practices throughout the AI development process. This includes utilizing distinct validation and test datasets to verify the models’ functionality under varying conditions, and implementing cross-validation techniques to assess the models’ accuracy.
>95% | >95% | >90% |
---|---|---|
Accuracy of verbal models | Accuracy facial expressions | Accuracy in predicting impressions |
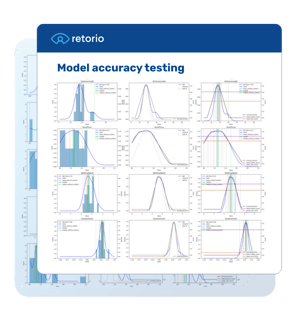
Our team: We are scientists at heart
After years of Ph.D. research in behavioral and differential psychology, we decided to make our insights available, equitable, and scalable to everyone. We employ psychologists, AI specialists, and business experts from more than a dozen countries.
Dr. Florian Abicht
Chief Data Science Officer
Florian serves as Chief Data Science Officer at Retorio, where he leads the AI team. Florian holds a Ph.D. from the Technical University of Berlin, conducted research across various prestigious German institutes, and worked in tech firms in the US and Germany before joining Retorio.
Dr. Christoph Hohenberger
Co-CEO & CPO
Christoph is a co-founder of Retorio and leads our product team. He holds a Ph.D. in psychology from the Technical University of Munich (TUM) with research at MIT, where he developed Retorio's Behavioral Intelligence models that power our AI coach.
Dr. Patrick Oehler
Co-CEO & CRO
Patrick is a co-founder of Retorio and leads Retorio's customer-facing teams. After studying Management at LSE, he obtained a Ph.D. in behavioral research from the Technical University of Munich (TUM). At TUM and Uni Tokyo, he researched the foundational models of AI coaching.
Based on our research studies at leading universities in the EU, USA, Japan, & UK.
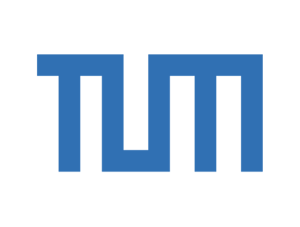
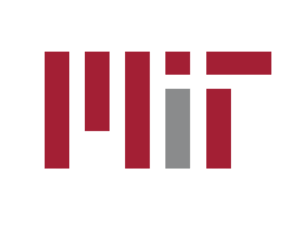
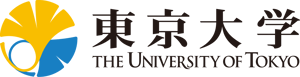
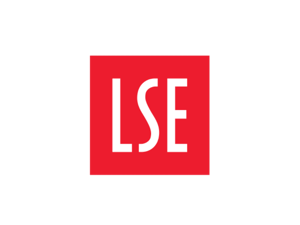
FAQs
We’re committed to ensuring the security and protection of the personal information that we process. We provide a GDPR-compliant and consistent approach to our data protection and only work with certified partners.
Retorio is a cutting-edge AI Coaching Platform that fuses machine learning with scientific findings from psychology and organizational research to ultimately take learning and development to a new level within organizations.
At the core of Retorio’s capabilities are its AI-powered immersive video simulations. Through these engaging role-plays, learners using Retorio get to train and develop the necessary skills through realistic scenarios. Furthermore, the personalized, on-demand feedback learners receive allows for immediate behavior change and performance improvement.
Retorio’s training platform transcends the limitation of scalability and redefines how individuals and teams train and develop, bringing talent development to a new dimension.
Retorio is used by organizations around the globe from < 20 people growth startups to global enterprises with up to 400.000 employees (you will find some of their logos on our website).
Moreover, we partner with selected HR consultancies, headhunters, and training agencies to serve our global audience. If you are interested in partnering, please contact us through our partner page.
We’re committed to ensuring the security and protection of the personal information that we process.
We are based in Germany and exclusively store data within the European Union.
We provide a GDPR-compliant and consistent approach to our data protection. Find out more about our Ethics and Privacy.
Retorio's AI Coaching Platform does not fall under the prohibited AI use cases under the AI Act, Chapter II, Article 5 or any other articles.
The EU AI Act explicitly addresses the use of emotion recognition systems and social scoring classifies them as prohibited AI applications when used in certain contexts. This regulation primarily targets AI systems that attempt to infer emotional states from biometric data such as facial expressions, body language, or physiological signals and scoring systems that aim at preventing individuals from getting access to certain resources.
While detecting emotions is forbidden by the AI Act, detecting readily apparent facial expressions, such as “smiling” is explicitly exempted from the list of prohibited use cases in Article 5 of the AI Act.
Technical Architecture The AI coaching system works through a combination of deep learning models designed to optimize descriptive, predictive, and prescriptive functions. These models are designed to recognize speech, voice and visual cues from video and audio data. The AI coaching platform consists of three primary types of models:
AI Coaching Generation and Simulation Models: These models generate realistic training content, including simulated conversations and avatars, enabling administrators to create and launch simulations rapidly. Generative AI creates coaching content and brings content to life.
AI Coaching Models for Behavioral Intelligence: These models analyze user interactions to quantify, predict, and prescribe behaviors using video analysis. These models translate explicit goals into concrete behaviors.
AI Coaching Impact Reporting Models: These models provide insights into user engagement and the overall impact of training, enabling administrators to evaluate the effectiveness of coaching initiatives. These models identify skill gaps and track coaching impact on the firm.
Quantifying Behavior with Behavioral Intelligence At the heart of Retorio's AI coaching platform is behavioral intelligence, which analyzes user interactions to quantify, predict, and prescribe behavior based on video analysis. This system employs multimodal models that operate across different layers, integrating voice, speed, and visual inputs to describe quantifiable behavioral traits.
Multimodal models capture verbal, visual, and auditory cues. Communication is 55% visual, 38% vocal, and 7% words, making multimodal models up to 14 times more powerful than pure verbal models. The data is processed through three layers:
Figure shows Three model layers of retorio AI coaching platform
Descriptive Recognition Models (Layer 1): In this initial stage, video and audio data are preprocessed and converted into quantifiable formats. Visual analysis involves detecting and analyzing visual cues like facial expressions using convolutional neural networks (CNNs). Verbal analysis employs deep-learning transcription models to extract audio features. Vocal analysis quantifies vocal data to enable prosody analysis.
Prediction Models (Layer 2): The data from Layer 1 is analyzed using specialized behavioral models that assess the anticipated impact of the observed behavior on a simulated target audience. Each model concentrates on a single mode of communication—vocal, verbal, or visual—to enhance explainability and transparency.
Prescriptive Benchmark Models (Layer 3): The output from Layer 2 is evaluated against predetermined benchmarks that represent ideal behaviors. The AI coach then compares observed behavior with these benchmarks and conducts a disparity analysis to determine necessary behavioral modifications, providing targeted recommendations.
Establishing Benchmarks Benchmarks reflect ideal behaviors, enabling progress tracking and discrepancy identification. Benchmarks can be derived through three methods:
Manual Configuration: Training administrators define benchmarks by outlining desired behaviors for specific situations.
Reference Benchmarks: Retorio offers pre-established benchmarks for various industries and roles, developed and maintained by behavioral scientists.
Benchmark Users: Top performers are invited to participate in training initiatives, and their behaviors are analyzed to create data-driven benchmarks.
Measuring Impact and Delivering Actionable Insights AI coaching impact reporting models provide insights into user engagement and training effectiveness. These models combine content generation outcomes with behavioral intelligence to evaluate coaching initiatives. The results are integrated into dashboards that help decision-makers monitor the efficacy of coaching programs. Dashboards offer metrics such as:
The number of users engaged and completion rates for individual coaching modules
Learning effectiveness of coaching programs
Business impact of coaching programs
By integrating with CRM or HRM systems, the platform can validate whether feedback results in actual performance improvement.
Key Advantages of Retorio's AI Coaching:
Data-Driven Recommendations: AI coaches offer recommendations supported by video evidence.
Impact Measurement: AI coaching quantifies behavioral changes and validates recommendations.
Unbiased Recommendations: AI coaches focus solely on behavior, disregarding demographics.
Data Privacy: Users have control over their data and can delete private information.
Psychological Safety: The AI offers a safe environment for receiving feedback.
Scalability and Democratized Access: Cloud-based AI coaching enables simultaneous coaching for many clients at low costs.
Statistics Retorio’s AI coaching models achieve high accuracy in describing and predicting behaviors: verbal and non-verbal accuracy both exceed 95%, while vocal accuracy is over 80%. Engagement rates are also high. Voluntary participation rates in AI coaching programs range from 75% (leadership training) to 93% (retail sales setting), with an average of six to twelve coaching sessions per user per quarter and recommendation rates of 80-91%. Participants' behavior improved by 2% with each AI coaching session. A large enterprise implemented Retorio’s AI coaching and within four months transitioned one out of five moderate performers into the top-performer category, resulting in an estimated 7% increase in additional revenue.